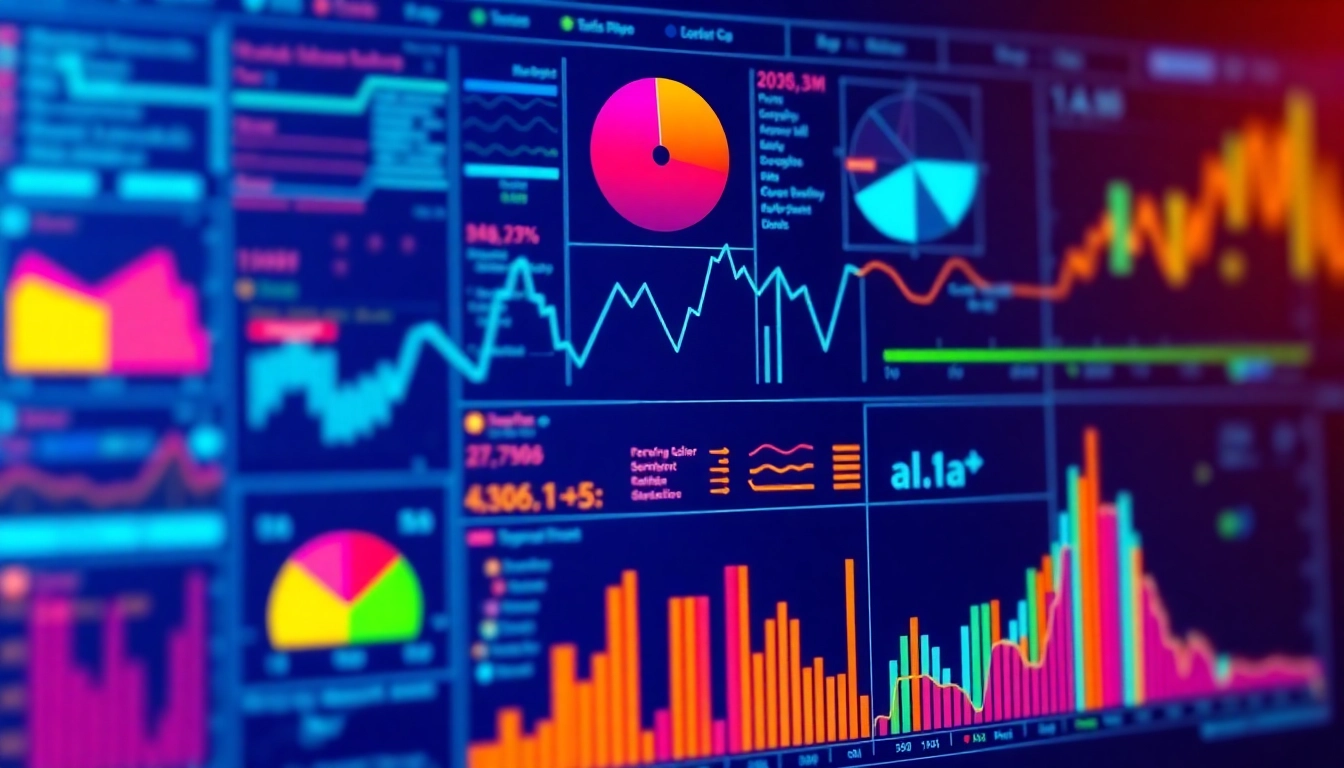
Enhancing Insights with AI Sentiment Analysis: A Comprehensive Guide
Understanding AI Sentiment Analysis
Definition and Importance
AI sentiment analysis refers to the method of using artificial intelligence technologies to interpret and classify emotions expressed in text. It operates at the intersection of natural language processing (NLP) and machine learning (ML) to extract subjective information from large volumes of data, such as social media posts, customer reviews, and feedback forms. The importance of sentiment analysis is underscored by its ability to provide organizations with actionable insights into public perceptions and emotional responses. By leveraging AI sentiment analysis, businesses can gain a strategic advantage, optimize their marketing efforts, and enhance customer relations.
How AI Sentiment Analysis Works
The process of AI sentiment analysis generally starts with data collection. Available textual data is gathered from various sources such as social media platforms, online reviews, blogs, and forums. AI models then employ natural language processing techniques to analyze this data. The analysis typically includes tokenization (breaking down text into words or phrases), part-of-speech tagging, and named entity recognition to identify key components of the text.
Once the data is pre-processed, sentiment classification can begin, with AI leveraging supervised, unsupervised, or semi-supervised learning techniques. Algorithms analyze the text through predefined rules or by learning patterns from previously labeled datasets. The end goal is to categorize sentiments into positive, negative, or neutral, providing a clear understanding of emotional tone for each piece of content analyzed.
Business Implications
AI sentiment analysis has substantial business implications. For instance, companies can gauge customer satisfaction in real-time, reacting swiftly to negative experiences. Moreover, businesses can identify trends in public sentiment towards their products or services, making it possible to tailor marketing strategies effectively.
Additionally, leveraging sentiment analysis can enhance product development cycles by interpreting customer feedback to make data-driven decisions. By examining sentiment trends over time, businesses can predict market behavior and customer needs, leading to competitive advantages.
Key Techniques in AI Sentiment Analysis
Natural Language Processing Approaches
Natural Language Processing (NLP) is the backbone of AI sentiment analysis. Techniques such as tokenization, stemming, and lemmatization play significant roles in parsing the text. Tokenization allows the system to break down sentences into individual units called tokens, which are easier to analyze. Stemming and lemmatization help normalize these tokens to their base form, improving the accuracy of sentiment analysis.
Advanced NLP approaches also involve the use of pre-trained language models like BERT (Bidirectional Encoder Representations from Transformers) and GPT (Generative Pre-trained Transformer). These models excel in capturing contextual nuances within text data, enhancing the accuracy of sentiment classification.
Machine Learning Algorithms
Machine learning algorithms play a pivotal role in sentiment analysis. Various algorithms, such as logistic regression, support vector machines (SVM), and Naive Bayes classifiers, are employed to classify sentiment accurately. More advanced methods involve deep learning techniques utilizing neural networks to uncover complex patterns within large datasets.
The choice of algorithm often depends on the size and complexity of the dataset. For extensive datasets, deep learning models are preferred due to their ability to learn from vast amounts of information and identify subtle sentiments.
Automated Sentiment Detection
Automated sentiment detection has revolutionized how businesses analyze text data by streamlining the process and allowing for real-time feedback. This automation is critical for organizations handling large volumes of information, as it drastically reduces the time and effort required for manual sentiment analysis.
Tools and platforms powered by AI enable businesses to automate sentiment detection across various channels, from customer service interactions to social media posts. Continuous learning algorithms refine sentiment analysis techniques over time based on feedback and new data, enhancing accuracy and relevancy.
Applications Across Industries
Marketing and Customer Insights
In the marketing realm, AI sentiment analysis offers profound insights into consumer behavior. By analyzing feedback across platforms, companies can develop targeted marketing strategies that resonate with their audience’s emotional responses. Understanding how customers feel about a brand can significantly influence marketing campaigns, product launches, and overall brand positioning.
Sentiment analysis can also be used to gauge customer responses to advertising campaigns, allowing companies to tweak or rethink their approaches based on public sentiment.
Product Feedback Analysis
AI sentiment analysis is essential in product management, providing companies with insights derived from customer feedback. By effectively assessing user sentiment concerning product features or usability, businesses can prioritize features in development, resolve pain points, and refine their product offerings. This strong feedback loop ensures customer expectations are met or exceeded, fostering loyalty and trust.
Social Media Monitoring
Social media represents a real-time pulse of public sentiment, making it a rich source of data for AI sentiment analysis. Companies use sentiment analysis to monitor brand mentions, measure the impact of campaigns, and track overall brand health. By understanding public emotion towards their brand, organizations can engage proactively with their audience and handle crises effectively.
Implementing AI Sentiment Analysis in Your Business
Choosing the Right Tools
When implementing AI sentiment analysis, selecting the right tools is critical for success. Various AI platforms and software offer sentiment analysis capabilities, each with its strengths. Consider factors such as ease of use, scalability, integration capabilities, and cost when choosing tools.
Popular tools include IBM Watson, Google Cloud Natural Language API, and proprietary platforms that offer custom solutions. Assessing the specific needs and technical capabilities of your business will guide you in selecting the most appropriate tool.
Establishing Best Practices
Establishing best practices in your sentiment analysis approach is vital for obtaining reliable results. Begin by ensuring data quality through proper data cleansing and pre-processing. Consistent labeling of training data is also essential for the accuracy of supervised models.
This includes defining clear criteria for positive, negative, and neutral sentiments to maintain uniformity across your data sets. Regularly updating your models with new data and feedback will ensure that your sentiment analysis remains relevant and reflective of current trends and sentiment shifts.
Measuring Performance and Impact
Measuring the performance and impact of AI sentiment analysis is crucial for demonstrating its value to your business. Key performance indicators (KPIs) such as accuracy, precision, recall, and F1 score can provide insights into model performance. Additionally, evaluating the outcomes of sentiment-based actions (i.e., marketing adjustments, product improvements) helps gauge the real-world impact of sentiment analysis initiatives.
Regular audits of sentiment analysis outcomes can also inform future strategy and help refine methods for better effectiveness over time.
Future Trends in AI Sentiment Analysis
Advancements in NLP Technology
The landscape of AI sentiment analysis is continually evolving, particularly with advancements in NLP technology. Future developments are likely to focus on improving the contextual understanding of language, enabling sentiment analysis tools to recognize sarcasm, cultural nuances, and emotional complexities more accurately.
Emerging methods in deep learning, such as transformer architectures and advanced embedding techniques, promise enhanced capabilities for understanding and interpreting user sentiment across diverse sectors.
Ethical Considerations
As with many AI technologies, ethical considerations in sentiment analysis are paramount. Issues surrounding privacy, data security, and the potential for misuse must be addressed. Organizations should strive for transparent practices regarding data usage and be clear with customers about how their information is analyzed.
Adopting ethical frameworks that guide the development and use of sentiment analysis technologies can help promote responsible AI usage and build public trust in these systems.
Integration with Other AI Applications
Integrating sentiment analysis with other AI applications will propel its capabilities even further. Combining sentiment analysis with chatbots and customer service platforms can enhance user interaction quality, providing responses based on individual customer sentiment.
Moreover, integrating sentiment analysis with predictive analytics can allow businesses to foresee market trends and changes in consumer sentiment, enabling proactive decision-making.