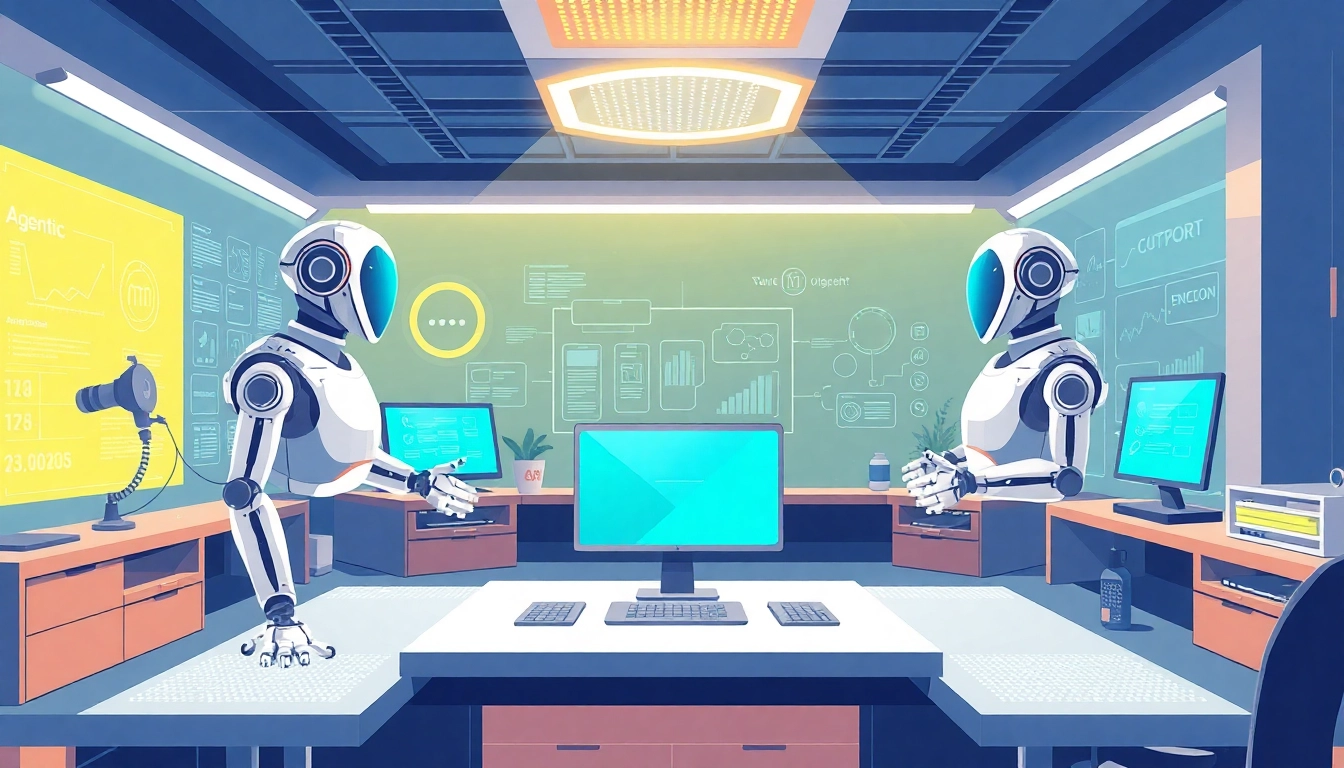
Harnessing Agentic Human AI: Transforming Autonomous Decision-Making in Modern Workspaces
Understanding Agentic Human AI
In the fast-paced world of artificial intelligence, the emergence of Agentic Human AI represents a significant stride towards developing autonomous systems capable of sophisticated decision-making. This innovative paradigm of AI transcends traditional machine learning models by empowering AI agents to not only process data but also act independently to achieve specific goals. This article delves into the definition, characteristics, historical context, and the transformation from conventional AI systems to agentic ones.
Definition and Key Characteristics
Agentic Human AI refers to AI systems endowed with a degree of agency—meaning they can autonomously execute tasks and make decisions without continuous human oversight. These systems are designed to analyze their environments, interpret data, set objectives, and choose actions based on those objectives. Key characteristics of agentic AI include:
- Autonomy: Agentic AI can operate independently, engaging in tasks without requiring human intervention.
- Adaptability: These systems can adapt to new information or changing conditions, allowing them to refine their actions and strategies over time.
- Goal-oriented Behavior: Agentic AI is programmed to perform specific tasks aimed at achieving defined goals, whether in data analysis, decision-making, or operational tasks.
- Complex Problem Solving: Utilizing advanced algorithms, agentic AI is capable of solving intricate, multi-step problems that may be beyond traditional AI’s capacity.
Historical Context and Evolution
The concept of agentic AI is rooted in decades of advancement within the field of artificial intelligence. Initially, AI systems were primarily rule-based, executing tasks as predefined by human programmers. This limited approach restricted flexibility and adaptability. With the advent of machine learning and neural networks, AI began to learn from data, but human oversight was still necessary.
As computational power surged and data availability expanded, researchers began exploring AI models that could learn autonomously and make decisions grounded in probabilistic reasoning. This laid the groundwork for the agentic models we see today. In recent years, developments in reinforcement learning and other advanced methodologies have propelled agentic AI, bringing it closer to operational real-world applications across diverse industries.
Comparison with Traditional AI Systems
To appreciate the advancements brought by agentic AI, we must compare it with traditional AI systems. Unlike traditional models that generally require explicit programming and continuous human monitoring, agentic AI embodies a paradigm shift by incorporating cognitive functions akin to human reasoning. Here are some notable distinctions:
- Decision-Making: Traditional AI systems rely heavily on pre-programmed rules, whereas agentic AI utilizes dynamic decision-making capabilities based on live data and context.
- Human Interaction: While traditional AI often necessitates human input for analysis or execution, agentic AI can operate independently, significantly reducing the need for human oversight.
- Adaptability: Agentic AI systems use feedback loops to adjust their behaviors and strategies on the fly, compared to the static nature of traditional algorithms, which require reprogramming for changes.
Applications of Agentic Human AI
The versatility of agentic AI allows for its applications across various sectors, from healthcare to finance, education, and beyond. Below, we examine some notable area implementations, backed by case studies and future potential.
Industry Implementations
One of the most compelling features of agentic human AI is its adaptability across various industries. Here are a few domains actively integrating this technology:
- Healthcare: Agentic AI is revolutionizing diagnostics and patient care by autonomously analyzing medical images and patient data to suggest treatment plans, enhancing the precision and speed of healthcare delivery.
- Finance: Financial institutions utilize agentic AI to detect fraud, optimize trading strategies, and assess creditworthiness. These systems can analyze vast datasets in real-time to make decisions that can lead to significant financial savings and improved compliance.
- Manufacturing: In the industrial sector, agentic AI can oversee production lines, predict machine failures, and optimize supply chain management, thereby increasing efficiency and minimizing downtime.
Case Studies and Success Stories
Numerous companies have harnessed the power of agentic AI to propel their operations forward:
- Healthcare Innovations: A healthcare startup developed an agentic AI platform that successfully diagnoses skin cancer based on image recognition, achieving an accuracy rate higher than most dermatologists.
- A Financial Breakthrough: A leading bank implemented an agentic AI system that accurately forecasted market trends, leading to a 20% increase in investment portfolio performance within a year.
Future Potential Across Sectors
The future implications of agentic AI are vast. As technology evolves, we expect to see synergies between AI agents and existing technologies, leading to enhanced efficiencies and capabilities. Future developments may include:
- Widespread use in sustainable practices, such as smart grid management and resource optimization.
- Collaborative robots (cobots) in manufacturing and service industries that work alongside humans while autonomously completing specialized tasks.
Benefits and Challenges
The embrace of agentic AI presents several advantages and challenges that organizations must navigate to fully leverage this technology.
Advantages of Implementing Agentic Human AI
Organizations that successfully integrate agentic AI can realize significant benefits:
- Increased Efficiency: By automating routine tasks and decision-making, agentic AI allows human workers to concentrate on more strategic initiatives, improving overall productivity.
- Enhanced Decision-Making: Agentic AI draws from real-time data to inform decisions, resulting in more informed and timely action.
- Cost Savings: Implementing agentic AI can reduce operational costs by minimizing human error and streamlining processes.
Common Challenges and Mitigation Strategies
Despite the advantages, organizations may encounter challenges including:
- Integration Difficulties: Merging new agentic AI systems with existing technologies can be complex. A phased rollout approach, supplemented with robust training programs, can ease this transition.
- Data Privacy Concerns: Handling sensitive data must be prioritized to maintain compliance with regulations such as GDPR. Organizations should implement strict data governance frameworks and encryption protocols.
Ethical Considerations in Autonomous Systems
As AI systems gain autonomy, ethical concerns arise around accountability, bias, and job displacement. It is crucial for organizations to establish ethical guidelines governing AI deployment, ensuring systems remain transparent, fair, and accountable for their actions.
Integration Strategies
Successful integration of agentic human AI into existing business frameworks requires thoughtful planning and execution. Here are key strategies to consider:
Steps to Incorporate Agentic Human AI into Your Business
The incorporation of agentic AI into your business can be navigated using the following steps:
- Assess Needs: Identify areas within your operations that could benefit from automation or better decision-making.
- Select Appropriate Tools: Choose AI platforms and tools that align with your strategic goals and operational needs.
- Pilot Testing: Conduct pilot studies to evaluate performance, gather feedback, and make adjustments before full implementation.
Interoperability with Existing Technologies
Ensuring that agentic AI systems seamlessly integrate with your existing technology stack is vital for operational cohesion. Companies should prioritize systems that offer interoperability features, allowing for data exchange and multi-system collaboration.
Monitoring and Governance Best Practices
Establishing a robust governance framework for agentic AI systems is essential for ongoing performance management:
- Regularly audit AI decisions to ensure they align with business objectives and ethical guidelines.
- Maintain transparency in how AI models operate, keeping stakeholders informed about potential biases and data sources.
The Future of Agentic Human AI
Looking ahead, the trajectory of agentic human AI promises to be transformative across various aspects of society and industry. Here, we explore anticipated developments and the evolving landscape of human-AI collaboration.
Trends and Predictions in AI Development
As technological capabilities expand, we foresee trends such as:
- A growing emphasis on ethical AI, integrating compliance and ethics seamlessly into AI systems.
- Increased collaboration among AI and humans, leveraging the strengths of each to achieve enhanced outcomes.
Continuous Learning and Adaptation
Ongoing advancements in machine learning will continually enhance agentic AI’s capability to learn from past experiences, adapt effectively to changes, and maintain relevance in dynamic environments.
Collaboration Between Humans and AI
Finally, the future of work will increasingly hinge on collaboration between agents and humans. As agentic AI systems take on more responsibilities, human workers will shift into roles that capitalize on creativity, empathy, and strategic thinking—qualities inherent to human nature.
In conclusion, the evolution of agentic human AI marks a pivotal chapter in technological advancements, poised to reshape industries and redefine human interactions with machines. Organizations that embrace this paradigm will not only enhance their operational capabilities but also position themselves at the forefront of innovation.