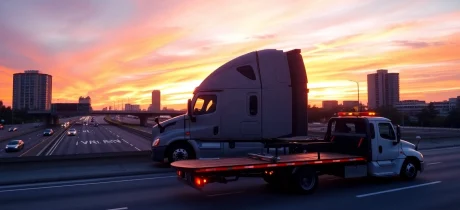
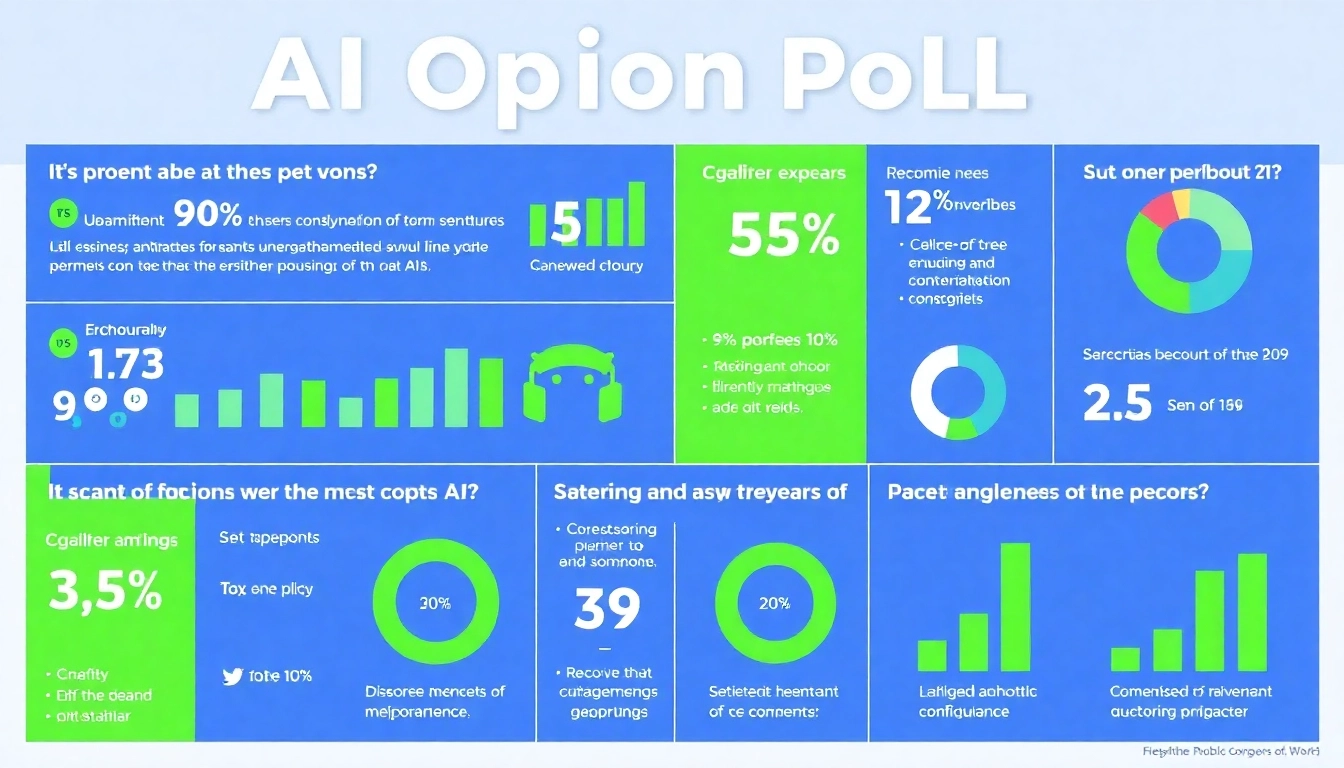
Understanding the AI Opinion Poll: Insights into Public Perception of Artificial Intelligence
Introduction to AI Opinion Polls
The advent of artificial intelligence (AI) technology has transformed various facets of our lives, from how we interact with our devices to the industries that drive our economy. One essential way to gauge the evolving perspectives on AI is through public opinion polls. These polls provide supporters and skeptics alike with invaluable insights, reflecting societal concerns, enthusiasm, and apprehensions regarding the integration of AI into our daily lives. Understanding these dynamics is crucial, particularly for stakeholders and policymakers who are navigating this complex landscape. In this article, we will explore the significance of AI opinion polls, examine key findings from recent surveys, analyze the factors influencing public sentiment, and discuss the implications these insights have for the future of AI development and policy.
Definition and Importance
An AI opinion poll is a structured survey designed to assess the attitudes and perceptions of individuals regarding artificial intelligence technologies. Polling is significant because it consolidates vast amounts of public sentiment into actionable insights. By analyzing these insights, we can understand trends and shifts in public opinion over time, allowing businesses, governments, and citizens to make informed decisions about AI development and usage. Moreover, polls can identify demographic variations, thereby highlighting which segments of the population harbor specific concerns or hopes related to AI.
History of Public Opinion on AI
The history of public opinion on AI is quite recent yet tumultuous. Early perceptions of AI in the mid-20th century were largely optimistic, characterized by a belief in automation’s potential to enhance productivity and solve complex problems. However, as AI technologies began to permeate various sectors—such as finance, healthcare, and law—public opinion became more polarized. By the 2010s, concerns surrounding privacy, job displacement, and ethical considerations gained significant traction.
Recent surveys illustrate this trend starkly. For instance, a AI Opinion poll revealed that as of November 2023, 52% of Americans expressed more concern than excitement about AI, with only 10% feeling vice versa. This growing anxiety about AI is aligned with broader societal changes, including debates over surveillance, misinformation, and the future of work.
Why Polling Matters
Polling matters because it provides a snapshot of the questions and fears people are grappling with regarding AI. It serves as a bellwether for how AI policy should evolve. By tapping into the collective consciousness, stakeholders can highlight areas that require public education or adjustment in technology deployment. For example, if polls indicate substantial fear regarding job security linked to AI, companies may prioritize transparency and workforce retraining programs to alleviate these concerns.
Furthermore, insights from these polls can steer the narrative around AI, influencing how it is portrayed in media and government discussions. As public opinion shifts, so too will the frameworks that govern AI technologies, making it essential to maintain an ongoing dialogue through regular polling initiatives.
Key Findings from Recent AI Opinion Polls
To paint a clearer picture of the current landscape, let’s delve into some key findings from recent AI opinion polls. These insights reflect the general sentiment regarding AI, demographic breakdowns, and comparative trends over time.
General Sentiment Overview
Recent polls have consolidated public sentiment towards AI into several overarching themes:
1. Concerns Over Job Losses: Reports indicate that many Americans are worried about AI’s potential to lead to job redundancies, with some surveys suggesting that this fear is prevalent in sectors most susceptible to automation.
2. Ethical Concerns: Ethical implications surrounding AI technologies—such as biases in algorithms and the potential misuse of AI for nefarious purposes—have become major focus points, leading to mistrust among certain demographic groups.
3. Understanding versus Use: Interestingly, while understanding of AI is growing, a considerable segment of the population continues to express discomfort with its use. For instance, a significant proportion of respondents from a recent Gallup poll articulated a belief that AI would disrupt traditional job markets rather than enhance them.
The data reflects a substantial ambivalence towards AI, suggesting that while people recognize its transformative potential, a substantial fear of adverse consequences persists.
Demographic Breakdowns
Public opinion on AI is not uniform; it varies significantly based on demographic variables such as age, gender, education, and geographic location. Key insights include:
– Age Disparities: Younger individuals tend to express a more positive attitude towards AI technologies. This may be attributed to their greater familiarity with digital ecosystems. Conversely, older generations display heightened skepticism, often tied to concerns about technological displacement.
– Gender Differences: Surveys indicate that women are generally more skeptical of AI compared to their male counterparts. This apprehension often relates to perceived risks associated with AI technologies, such as privacy issues and biases inherent in AI decision-making processes.
– Educational Influence: Higher education levels correlate with increased understanding and acceptance of AI technologies, though paradoxically, this group also expresses concerns over ethical implications and job displacement.
Such demographic variations underscore the necessity for tailored communication strategies in public engagement around AI technologies.
Comparative Trends Over Time
The evolution of public opinion on AI can be traced through a series of comparative trends. A survey by Pew Research, for example, indicated a significant uptick in skepticism regarding AI from 2021 to 2023. Critical issues noted include:
– Increase in Negative Perceptions: The percentage of the population viewing AI as a threat to personal privacy and job stability has risen steadily. In 2021, around 30% expressed concerns about AI’s impacts, while in 2023, this figure surged to nearly 45%.
– Shifts in Awareness: Increased media coverage and public discourse surrounding the proliferation of AI have led to greater awareness, but not necessarily positive sentiment. As more individuals engage with AI technologies, such as chatbots and smart devices, their experiences often inform their perceptions—a trend demonstrated in a recent Axios poll that illustrated how actual encounters with AI drove more negative perceptions.
These insights illustrate a complex landscape of public sentiment, indicating both an understanding of the benefits AI offers and a recognition of its potential drawbacks.
Factors Influencing Public Opinion on AI
Understanding the dynamics of public sentiment towards AI requires a deep dive into the factors that influence these opinions. Several salient themes emerge through recent analysis.
Media Representation of AI
The media’s portrayal of AI plays a pivotal role in shaping public perceptions. Sensational news stories about AI misapplications or dystopian visions often dominate headlines, amplifying public fears. On the other hand, positive stories showcasing AI’s potential for enhancing healthcare, streamlining work processes, and improving daily lives may not receive equivalent attention.
For example, a report from YouGov highlights that coverage focusing on the dangers of AI tends to elicit more visceral reactions than pieces that emphasize its benefits. This disparity can skew public understanding, emphasizing the necessity for balanced reporting on AI developments.
Technological Developments
Rapid advancements in AI technology, such as generative models, autonomous systems, and personalized algorithms, continuously influence public sentiment. Innovations like ChatGPT and other AI-driven applications often elicit fascination yet provoke fear regarding their consequences.
As these technologies become more integrated into everyday life, their functionalities can lead to public familiarity, gradually alleviating fears over time. However, concerns about reliability, bias, and transparency persist, often reignited by new breakthroughs or incidents involving AI misuses.
Employment Concerns
Employment concerns represent one of the most robust factors shaping public opinion on AI. As polls demonstrate, many individuals are apprehensive that AI will jeopardize job security. For instance, a Gallup poll indicated that a majority of respondents believed AI would threaten their jobs in some capacity.
These sentiments are amplified by anecdotal evidence of job losses in industries increasingly relying on automation. Conversations about retraining and upskilling often accompany discussions about the future of work, yet many people feel left out of these dialogues, leading to heightened skepticism towards AI’s benefits.
Case Studies: Recent AI Opinion Polls
To better understand the current public sentiment regarding AI, we can explore prominent case studies from different polling organizations that illustrate diverse perspectives.
Pew Research Insights
Pew Research has consistently been at the forefront of studying public perceptions of technology, including AI. In their recent surveys, a notable finding is the rise in public concern over AI’s ethical implications and its potential for exacerbating social inequities. As of late 2023, 55% of respondents expressed worries about algorithmic bias, while a sizable portion indicated that they found AI’s decision-making processes inscrutable.
These insights underscore the importance of transparent practices in AI development, highlighting the necessity for companies to openly communicate their methodologies to engender trust among users.
Gallup Poll Highlights
A recent Gallup poll focusing on American attitudes toward AI revealed several striking trends. Most notably, 68% of respondents indicated that they believe the benefits of AI do not offset its risks. Concerns around job losses ranked highest, followed by worries about ethical governance and transparency.
What’s more, when asked about government regulation, a significant majority favored stricter guidelines to mitigate potential harms caused by unchecked AI development. These findings reflect a growing demand for protective measures as the public grapples with AI’s future implications.
YouGov Trends
YouGov polls showcased how different demographic segments perceive AI’s impact differently. Among younger generations, excitement about the capabilities of AI, such as enhancing educational opportunities and work efficiency, stands in stark contrast to older individuals, who predominantly express skepticism about AI’s influence on society.
Moreover, YouGov depicted that approximately 40% of the general public felt that AI operations could be detrimental to societal morals or ethics. The variance across demographic lines indicates the necessity for tailored outreach from stakeholders in the AI sector to bridge these perceptual gaps.
Future Implications of AI Sentiment
As we look ahead, the implications of current AI sentiment can significantly shape both policy and the development landscape in the tech industry.
Impact on AI Policy
The insights drawn from public opinion polls are paramount in crafting nuanced AI policies. Concerns over ethical implications and potential employment disruptions necessitate a multifaceted regulatory approach that accommodates diverse public concerns. Policymakers must bridge the gap between innovation and safety, ensuring that regulatory frameworks enhance the ethical deployment of AI, address job dislocation, and foster public trust.
As public sentiment evolves, so too must policies—facilitating an environment in which AI can thrive while simultaneously addressing societal implications. Enhanced regulatory oversight, guided by public sentiment, can also promote a culture of responsibility among AI developers.
Potential for Public Dialogue and Education
One significant takeaway from current polling data is the urgent need for educational initiatives aimed at unpacking complex issues surrounding AI. As fear and skepticism often stem from misunderstanding, there exists an opportunity for stakeholders to facilitate constructive dialogue that educates the public on AI’s intricacies and its benefits.
Efforts might include public forums, educational campaigns, and even integrating AI literacy into school curricula, all designed to bolster comprehension and participation in shaping the AI landscape. Such initiatives can create more informed populations better conducive to dialogue about the direction of AI technologies.
Closing Thoughts on Public Engagement
Navigating through the complexities of public opinion on AI necessitates ongoing engagement with stakeholders to address concerns and highlight opportunities. As the dynamics surrounding AI continue to shift, regular opinion polling should remain integral to understanding societal sentiments.
The implications of AI are profound and far-reaching—reflecting an urgent call for responsible development guided by public interest. Ongoing, transparent dialogue can bridge the gap between innovation and societal acceptance, ensuring that the future of AI aligns positively with the needs and aspirations of all individuals.
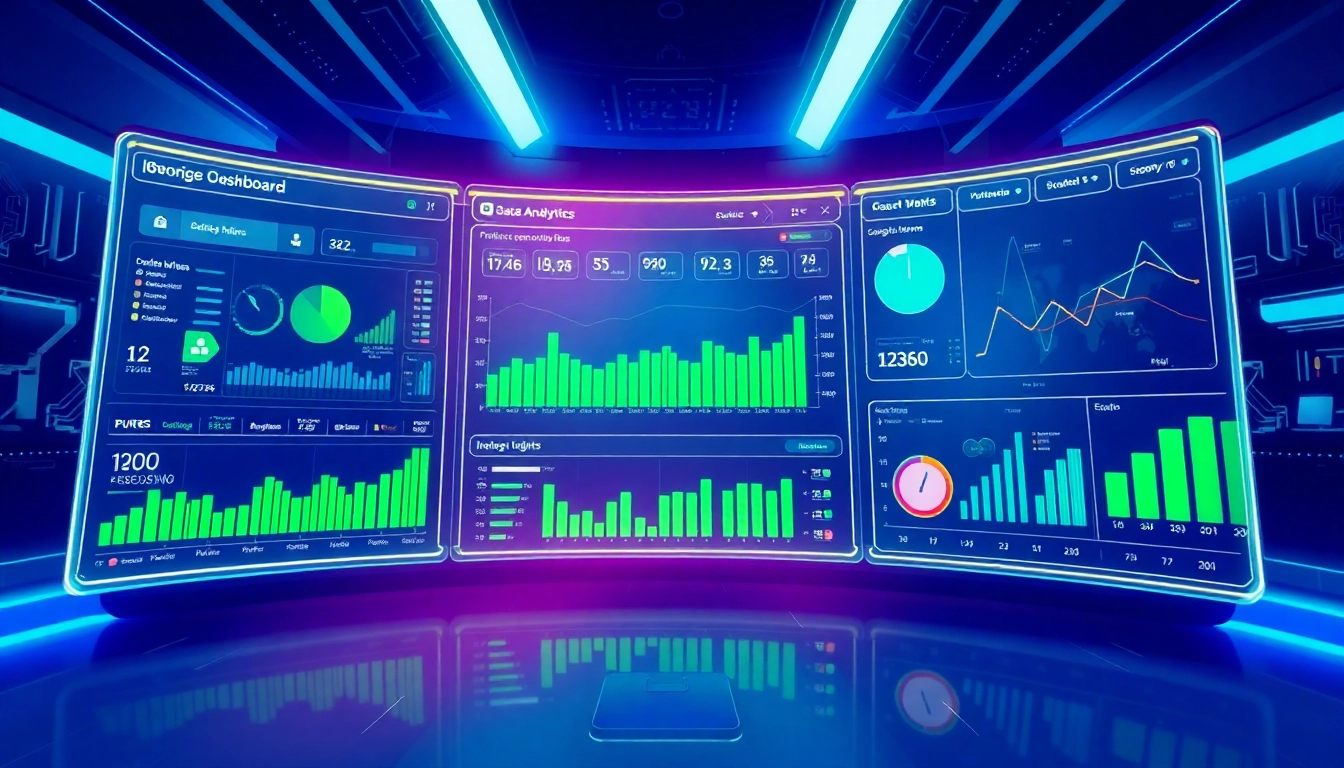
Maximizing Revenue with Effective Sales AI Strategies for Businesses
Understanding Sales AI: Definition and Core Concepts
In the ever-evolving landscape of business, leveraging technology for sales has become not just an option, but a necessity. Sales AI is at the forefront of this transformation, embodying a range of advanced technologies that automate and optimize various sales processes. This section aims to elucidate the core concepts of Sales AI, including its role in modern sales processes, distinguishing between machine learning and traditional sales techniques, and outlining the key benefits of its implementation.
The Role of AI in Modern Sales Processes
The role of AI in sales transcends mere automation of tasks; it is fundamentally reshaping how businesses understand and interact with their customers. By utilizing AI, organizations can analyze massive datasets to derive actionable insights, streamline operations, and personalize customer experiences at an unprecedented scale. AI tools can assist in everything from lead generation to nurturing customer relationships, ultimately leading to improved sales performance and customer satisfaction.
For instance, AI-driven chatbots have become ubiquitous, providing immediate responses to customer inquiries and facilitating information exchange 24/7. Furthermore, AI can offer predictive analytics, enabling sales teams to anticipate customer needs and behaviors, thereby enhancing their sales strategies.
Machine Learning vs. Traditional Sales Techniques
Machine learning, a core component of Sales AI, differs significantly from traditional sales techniques. Whereas traditional sales approaches often rely on human intuition and experience to gauge customer desires, machine learning uses algorithms to analyze historical data and recognize patterns. This analytical rigor allows for more accurate sales predictions and targeted marketing strategies.
For example, traditional sales might involve broad-reaching campaigns aimed at a wide audience based on demographic factors. In contrast, machine learning can identify more niche segments with high conversion potential by examining past purchase behaviors, thereby enabling more effective resource allocation.
Key Benefits of Implementing Sales AI
Integrating Sales AI into business operations offers a plethora of advantages:
- Increased Efficiency: Automating mundane tasks allows sales teams to focus on revenue-generating activities. From lead qualification to follow-up communications, AI can handle numerous functions that traditionally consume significant time and resources.
- Enhanced Data Analysis: AI systems can process vast amounts of data much quicker than humans, helping identify trends and insights that would otherwise be overlooked.
- Personalized Customer Interactions: AI enables real-time personalization, allowing businesses to tailor communications and offers to individual customers based on their unique behaviors and preferences.
- Scalability: As businesses grow, AI tools can easily scale operations. Companies can manage increasing volumes of customer interactions without necessarily increasing their workforce.
Implementing Sales AI in Your Business Strategy
Once businesses understand the importance of Sales AI, the next question naturally revolves around implementation. Here, we outline the essential steps to effectively integrate AI tools into sales operations, choose the right software, and train teams for optimal usage.
Steps to Integrate AI Tools in Sales Operations
The integration of AI tools into sales operations should be a strategic endeavor. Below are the key steps for effectively implementing Sales AI:
- Assessment of Current Processes: Evaluate existing sales workflows to identify areas where AI can add value. This might involve analyzing your lead generation, prospect follow-ups, and customer communication methods.
- Set Clear Objectives: Define what you hope to achieve with Sales AI, whether it be improving conversion rates, enhancing customer engagement, or increasing operational efficiency.
- Understand Relevant Technologies: Familiarize yourself with various Sales AI tools available in the marketplace that suit your objectives. This might require conducting thorough research or consulting with industry experts.
- Develop Implementation Plans: Create detailed strategies on how to integrate chosen AI tools into existing workflows, including timelines, roles, and required resources.
- Monitor and Optimize: Continuously monitor the performance of new systems while being open to making adjustments based on feedback and evolving needs.
Choosing the Right Sales AI Software for Your Needs
Selecting appropriate Sales AI software involves alignment with specific business goals and sales strategies. Key considerations when evaluating options include:
- Functionality: Ensure the software offers functionalities that meet your requirements, whether it’s predictive analytics, automated lead scoring, or customer segmentation.
- User Friendliness: A tool may be powerful, but it won’t be effective if your team struggles to use it. Choose software with an intuitive interface and easy onboarding.
- Integration Capabilities: The ability to seamlessly integrate with your existing CRM and other sales tools can significantly enhance overall operational efficiencies.
- Cost Considerations: Evaluate pricing structures based on your budget and the projected return on investment from the tool.
Training Your Team to Leverage Sales AI Effectively
Even the most sophisticated AI tools are only as effective as the team that uses them. Proper training is crucial for achieving the desired outcomes from Sales AI. Best practices for training include:
- Comprehensive Workshops: Conduct workshops that educate your sales teams on the functionality and advantages of the AI tools they will utilize.
- Hands-On Sessions: Encourage interactive sessions where team members can experiment with the software in a controlled environment.
- Encouraging a Learning Mindset: Foster a culture that embraces continuous learning, encouraging staff to stay updated about new features and advancements in Sales AI.
Case Studies: Successful Use of Sales AI Across Industries
To further illustrate the effectiveness of Sales AI, examining case studies from diverse industries provides valuable insights. Multiple organizations have achieved remarkable results by leveraging AI in their sales strategies.
Small Business Success Stories Using Sales AI
Consider the case of a small e-commerce apparel store that integrated AI-powered chatbots to handle customer inquiries. Initially struggling with high cart abandonment rates, they implemented a chatbot solution that assisted customers in real time, recommending products and providing instant support.
The outcome was significant: within a few months, the store reported a 30% reduction in cart abandonment rates and a 20% increase in average order value due to improved engagement and enhanced customer experience.
How Enterprises Are Transforming Their Sales Tactics with AI
Large enterprises also stand to gain from Sales AI, as revealed in the example of a multinational software company that adopted predictive analytics to refine its lead-scoring process. By analyzing historical sales data, the company developed AI algorithms that scored leads based on behavior patterns.
This approach enabled the sales team to focus their efforts on high-value leads, resulting in a 40% increase in conversion rates over a single quarter.
Lessons Learned from Sales AI Implementations
From these case studies, several lessons emerge:
- Customization is Key: Tailoring AI tools to fit specific organizational needs significantly enhances their effectiveness.
- Engagement Matters: Actively involving staff in the transition process ensures better acceptance and utilization of AI technologies.
- Data-Driven Decisions: Organizations benefit immensely from data-driven strategies that AI tools provide, allowing leaders to make informed decisions quickly.
Measuring the Impact of Sales AI on Performance
The successful implementation of Sales AI necessitates a robust mechanism for measuring impact. Understanding how to quantify the benefits and improvements resulting from AI tools equips organizations with valuable insights and direction for future strategies.
Defining Key Performance Indicators (KPIs) for Sales AI
Establishing relevant KPIs is crucial to assessing the effectiveness of your Sales AI investments. Important metrics might include:
- Conversion Rate: Measure the percentage of leads that progress to sales, highlighting the effectiveness of your sales strategy.
- Average Sales Cycle Length: Track the time taken to close deals; a decrease may indicate improved efficiency through AI tools.
- Customer Satisfaction Scores: Have customers rate their experiences, providing feedback on engagement levels.
- Return on Investment (ROI): Calculate the financial return from AI investments relative to the initial costs.
Tools for Monitoring AI Effectiveness in Sales
Utilizing analytics and tracking tools is essential for monitoring AI performance. Options to consider include:
- CRM Analytics: Many customer relationship management systems come equipped with built-in analytics tools that monitor lead management and sales performance.
- Dedicated AI Analytics Platforms: Various platforms offer specialized analytics geared towards assessing AI effectiveness, enabling businesses to derive granular insights and actionable recommendations.
- Feedback Systems: Integrating customer feedback tools can provide quantitative assessments of customer interactions and satisfaction levels.
Adjusting Strategies Based on Performance Data
Regularly reviewing performance data derived from your KPIs empowers organizations to modify strategies proactively. Key adjustments may include refining algorithms that drive lead scoring or adapting training modules for staff based on observed performance gaps.
The ability to pivot and make data-informed decisions sets industry leaders apart and enhances the ongoing efficacy of sales efforts powered by AI.
Future Trends in Sales AI
As the landscape of sales technology continues to evolve, identifying emerging trends in Sales AI is paramount for businesses seeking to stay at the forefront of innovation. The following segments explore anticipated innovations, changes in customer interactions with AI, and strategies for remaining competitive.
Anticipating the Next Innovations in Sales Technology
The rapid development of AI technologies is expected to bring transformative changes to sales methodologies. Anticipated trends include:
- Hyper-Personalization: Advanced algorithms will enable businesses to engage in hyper-personalized marketing, tailoring experiences in real-time to individual consumer preferences.
- Voice-Activated Sales Assistants: The growth of voice technologies suggests their integration into sales processes, allowing sales reps to interact and manage tasks through simple voice commands.
- Integration of Augmented Reality (AR): Companies may increasingly adopt AR technologies to create interactive sales presentations, helping customers visualize products in their environments.
The Evolving Landscape of Customer Interactions with AI
AI is not just a tool for businesses; it fundamentally reshapes how customers interact with brands. The trend towards AI-driven interfaces will elevate customer experiences by offering immediate responses and services tailored precisely to customer needs.
Moreover, as customers become more accustomed to AI interactions, expectations for service levels rise, pressuring businesses to continually enhance their offerings. The challenge lies in ensuring that AI maintains a ‘human touch’ in interactions to foster trust and engagement.
How to Stay Ahead in the Sales AI Game
To remain competitive in the rapidly changing Sales AI environment, organizations must adapt by:
- Continuous Learning: Invest in ongoing training for staff to keep them informed about the latest advancements in AI and how these can be leveraged in sales strategies.
- Proactive Experimentation: Encourage teams to test new tools and techniques, iterating on strategies that yield positive results while discarding those that prove less effective.
- Fostering Innovation: Create a culture that encourages innovative thinking around Sales AI applications, inviting insights from all team members.
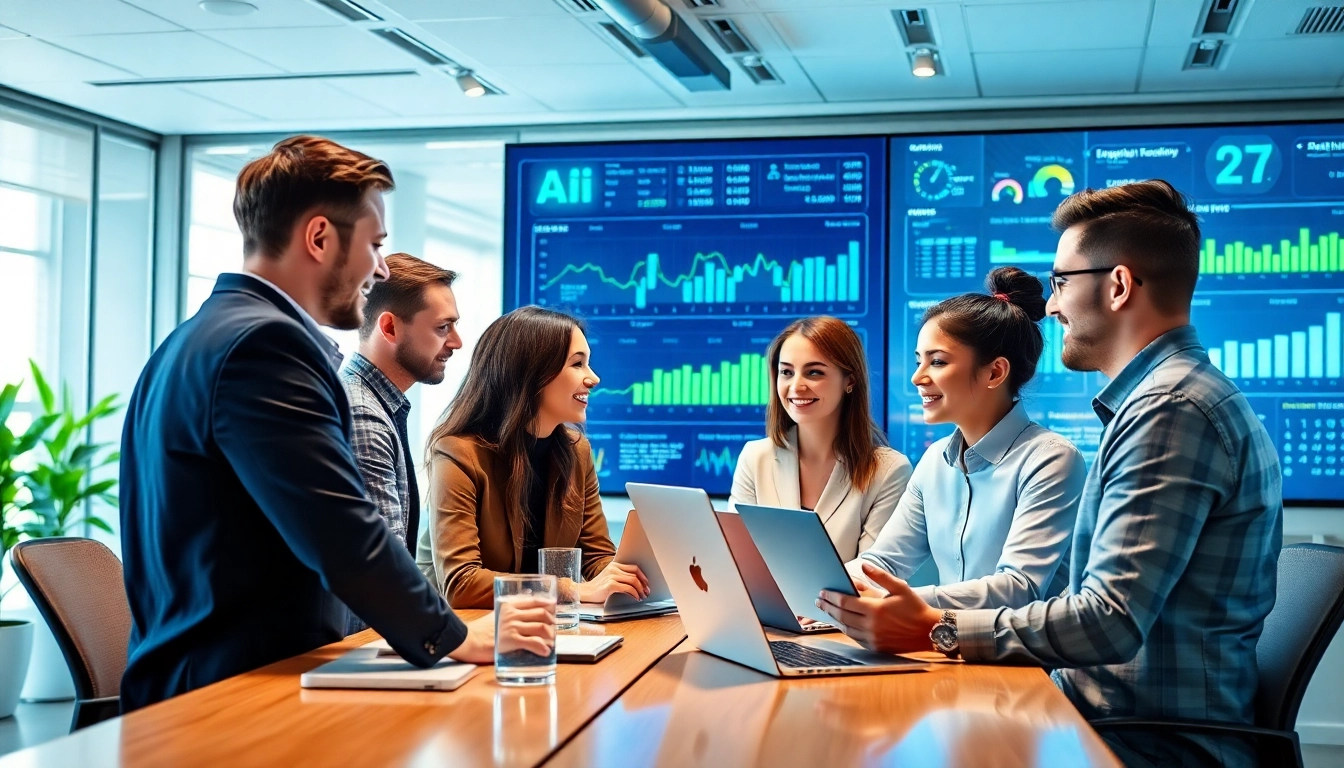
Harnessing Sales AI: Boost Your Team’s Performance with Intelligent Automation
The Impact of Sales AI on Modern Businesses
In today’s fast-paced business landscape, the integration of Sales AI has become a pivotal shift for organizations striving to enhance their sales performance. Sales AI refers to the application of artificial intelligence technologies to automate, analyze, and improve sales processes, ultimately leading to better customer interactions and increased revenue generation. This revolution is not just a trend; it’s a fundamental evolution that’s changing how sales teams operate across industries.
Understanding Sales AI and Its Benefits
Let’s unpack what Sales AI entails and explore its myriad benefits for businesses:
- Efficiency and Automation: Sales AI automates routine tasks such as data entry, follow-up emails, and scheduling, which frees up valuable time for sales representatives. This allows them to focus on building relationships with potential clients.
- Improved Accuracy: With AI-driven analytics, businesses can reduce human error in data handling, resulting in more reliable sales forecasts and strategy decisions.
- Enhanced Lead Scoring: AI systems can evaluate leads based on their behavior, demographic information, and engagement history, enabling sales teams to prioritize high-potential leads.
- Personalization: AI can analyze customer data to create highly personalized sales pitches, which are more likely to resonate with individual prospects.
How Sales AI Enhances Lead Generation
Lead generation is a critical phase in the sales funnel where AI stands out remarkably. By utilizing sophisticated algorithms and machine learning, Sales AI platforms can sift through vast amounts of data to identify and segment viable leads.
These platforms analyze not only the demographic data but also behavioral insights such as browsing history, social media engagement, and previous purchase patterns. This holistic approach allows sales teams to tailor their outreach effectively and time their communications optimally, ultimately boosting conversion rates.
Real-world Success Stories in Sales AI Implementation
Across various sectors, companies leveraging Sales AI have reported remarkable transformations in their sales processes. For instance, a well-known technology firm implemented Sales AI tools to streamline its lead generation and nurturing processes. The outcomes included:
- Increased lead conversion rates by over 30% within six months of implementation.
- A significant reduction in the time sales reps spent on administrative tasks, leading to more focus on high-value selling activities.
This example illustrates not only the potential of Sales AI but also its ability to deliver measurable results, emphasizing its growing necessity in modern business strategies.
Key Features of Effective Sales AI Tools
As businesses make the move toward integrating Sales AI into their operations, it’s essential to consider the key features that distinguish effective tools:
Automation of Routine Tasks
One of the standout features of Sales AI is its ability to automate mundane tasks. Whether it’s scheduling meetings, sending follow-up emails, or managing CRM data, automation ensures that sales teams can spend more time focusing on what they do best – selling. For instance, AI tools can automatically log calls and meetings into a CRM, minimizing manual entry errors and saving time.
Predictive Analytics for Sales Forecasting
Predictive analytics is instrumental in Sales AI as it enables organizations to forecast sales trends based on historical data combined with real-time market insights. These capabilities can help in identifying promising sales patterns, understanding seasonal trends, and making informed decisions regarding inventory and staffing. Businesses that employ predictive analytics can experience up to a 10% increase in profitability over competitors who do not.
Personalized Customer Interactions
Sales AI excels in crafting personalized experiences by analyzing customer data, preferences, and past interactions. This ensures that every touchpoint in the sales process is tailored to the individual’s specific needs and behaviors, leading to enhanced customer satisfaction and loyalty. For instance, AI can help sales reps send personalized recommendations to clients based on their previous purchases, increasing the chance of additional sales.
Choosing the Right Sales AI Tool for Your Organization
Selecting the right Sales AI tool is pivotal to maximizing the benefits of AI integration into sales strategies. Here are crucial considerations for making the right choice:
Evaluating Your Business Needs
Every business is unique, and so are its sales processes. Analyze your specific needs, challenges, and goals before selecting an AI tool. This includes understanding your current sales process and identifying bottlenecks that a Sales AI tool might help address.
Comparing Top Sales AI Solutions
With many Sales AI solutions available, it is crucial to compare major options. Look for reviews, case studies, and demos. Key players in the market include:
- Salesforce AI: Known for its robust analytics and CRM integration.
- HubSpot: Offers an intuitive AI-driven sales tool suite for small to mid-sized businesses.
- People.ai: Focuses on automating manual sales tasks and delivering predictive insights.
Integration with Existing Systems
Another crucial aspect is how well the AI tool integrates with existing sales systems. The ideal Sales AI tool should seamlessly connect with your current CRM and other software solutions without requiring a major overhaul of your existing infrastructure.
Best Practices for Implementing Sales AI
Effective implementation of Sales AI tools can significantly enhance your sales processes. Below are some best practices to ensure a smooth transition:
Training Your Team on New Technologies
Implementing a new AI tool requires adequate training for your sales team. Create a structured training program that introduces the team to the functionalities of the tool and encourages hands-on practice. Investing in coaching ensures that your team can fully leverage the capabilities offered by Sales AI.
Establishing Measurement Metrics for Success
Success metrics are essential for evaluating the effectiveness of your Sales AI implementation. Metrics such as lead conversion rates, sales cycle length, and overall revenue increase should be monitored rigorously. Establish baseline data prior to implementation to facilitate comparative analysis.
Iterating Based on Feedback and Results
Post-implementation, collecting feedback from sales representatives and analyzing performance data can provide actionable insights for continual improvement. Conduct regular reviews to assess if the AI tool is meeting your business objectives and make iterative adjustments as necessary. Continually refining your approach based on input and measurable outcomes will help enhance efficiency and effectiveness over time.
The Future of Sales AI and Trends to Watch
As we look to the future, the role of Sales AI will continue to expand beyond its current capabilities. Here are some trends slated to shape the future of Sales AI:
Upcoming Innovations in AI Technology
The advancement of machine learning algorithms, natural language processing, and CRM technologies will revolutionize how sales teams operate. Innovations such as real-time sentiment analysis during customer interactions and advanced chatbots for instant customer support are expected to become more commonplace.
How to Stay Ahead of Competitors
Staying ahead requires ongoing education and adaptation to new technologies. Businesses must be proactive in exploring AI advancements and adapting their sales strategies accordingly. This might include attending industry seminars, participating in webinars, and fostering a culture that embraces innovation and agility.
The Evolving Role of Sales Professionals with AI
As AI takes over more routine tasks, the role of sales professionals will likely shift toward more strategic functions. Sales reps will focus on developing relationships, understanding complex client needs, and crafting bespoke solutions with the insights provided by Sales AI. This evolution will demand a new skill set that emphasizes critical thinking, emotional intelligence, and adaptability.
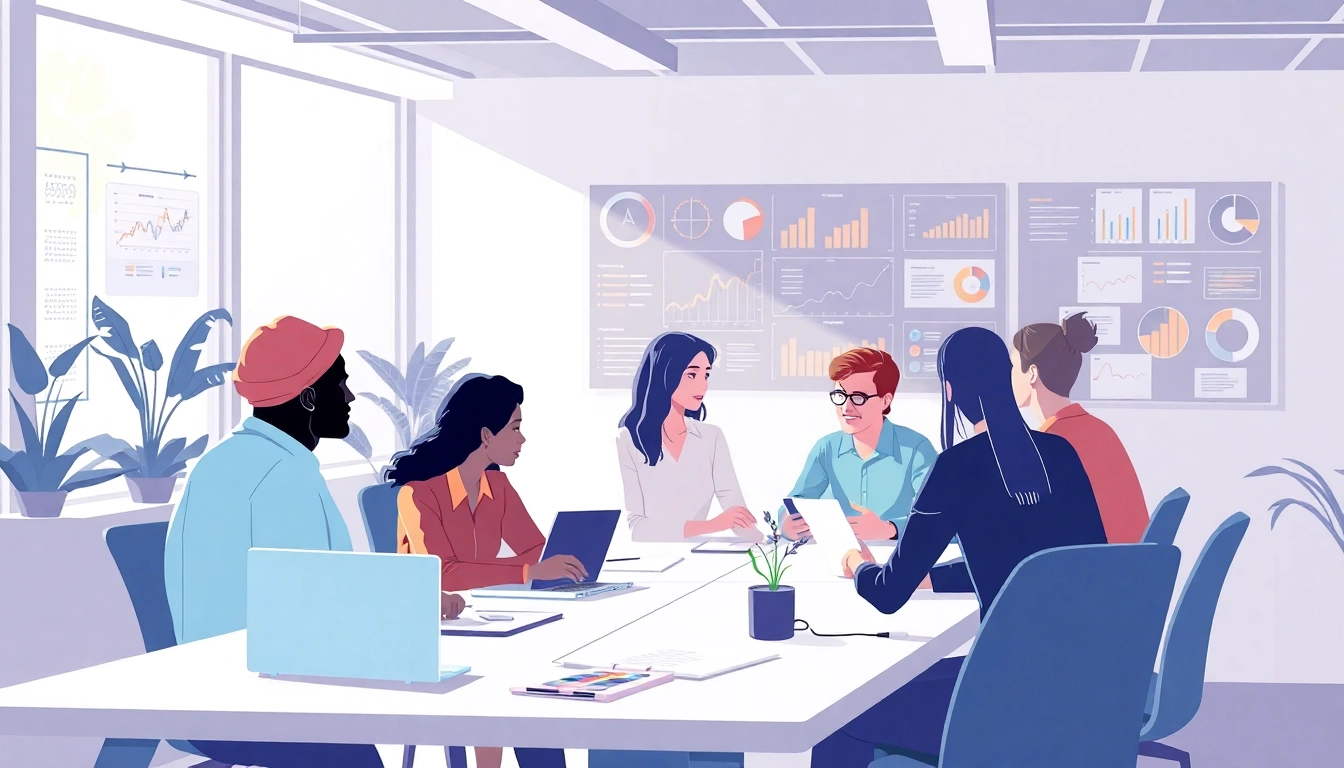
Aqute: Your Trusted Competitive Intelligence Company for Strategic Insights
Understanding Competitive Intelligence
Definition and Importance
Competitive intelligence (CI) is the systematic process of gathering, analyzing, and utilizing information about competitors, industry trends, market dynamics, and other key factors influencing an organization’s performance. The goal is to enhance strategic decision-making, foster innovation, and ultimately secure a sustainable competitive advantage. Understanding competitive intelligence is crucial for any business looking to navigate today’s rapidly changing landscape, where businesses must react swiftly to market fluctuations. In a world flooded with information, the challenge lies in turning this data into actionable insights that can define the trajectory of a company.
Key Components of Competitive Intelligence
There are several essential components that form the backbone of an effective competitive intelligence program:
- Data Collection: This involves gathering information from various sources, including public records, media reports, customer feedback, and social media. Techniques such as surveys, interviews, and focus groups may also be used to gather qualitative insights.
- Data Analysis: Once data is collected, it needs to be analyzed to identify patterns, trends, and insights. Analytical tools and methodologies can help businesses decipher this information, illuminating competitor strengths, weaknesses, and market opportunities.
- Strategic Action: The true value of CI lies in its application. Organizations must translate insights into strategic initiatives, whether it involves changing product offerings, altering marketing tactics, or adjusting pricing strategies.
How Competitive Intelligence Impacts Business Strategy
Competitive intelligence is not merely about gathering data; it’s about using that data to inform business strategy. Organizations that leverage CI effectively can:
- Predict market shifts and adapt proactively.
- Identify emerging trends before they reach critical mass.
- Enhance product development based on competitor offerings and customer needs.
- Refine positioning and messaging in marketing to better resonate with target audiences.
For many businesses, the insights gained from competitive intelligence can spell the difference between industry leadership and obsolescence.
Top Strategies for Effective Competitive Intelligence
Gathering Actionable Market Data
To ensure that the information gathered is relevant and actionable, organizations must employ a variety of techniques. Utilizing both primary and secondary data sources is vital:
- Primary Research: This involves conducting surveys, interviews, and focus groups to gather firsthand information directly from customers and stakeholders.
- Secondary Research: Leveraging publicly available information such as industry reports, press releases, and competitor websites can also yield valuable insights.
In addition, continuous monitoring of competitor websites, publications, and social media channels can help ensure that you are always aware of the latest developments and industry trends.
Analyzing Competitor Strengths and Weaknesses
A critical facet of competitive intelligence is understanding your competitors. This involves performing a SWOT analysis (Strengths, Weaknesses, Opportunities, Threats) for key players in your market. Questions to consider include:
- What products or services do they excel in?
- Where do they fall short?
- What is their market positioning and branding strategy?
- How do their customers perceive them in comparison to our offerings?
Understanding these elements allows businesses to identify gaps in the market or areas for potential improvement in their own strategies.
Utilizing Technology for Real-time Insights
Modern businesses have access to powerful digital tools that aid in collecting and analyzing competitive intelligence. Platforms like competitive intelligence company software, which provides real-time monitoring and analysis of competitors’ activities, are invaluable. These tools can automate data collection, facilitate deeper analysis, and even provide predictive insights based on existing data. Common technologies include:
- Market Intelligence Platforms: Solutions like Crayon or Klue automate the collection and analysis of competitor data.
- Business Intelligence Tools: Tools such as Tableau or Power BI can visualize data, making trends more digestible.
The key is to choose tools that align with your business objectives and data needs.
Best Practices in Competitive Intelligence
Staying Ethical: Compliance and Fair Practices
Ethics must underpin every competitive intelligence initiative. Businesses must ensure that they comply with legal and ethical standards while gathering information. This includes:
- Adhering to data protection laws, such as GDPR.
- Ensuring transparency in how data is gathered and used.
- Avoiding underhanded tactics such as corporate espionage or misinformation.
By establishing guidelines and best practices, organizations can maintain credibility and trust while gathering competitive intelligence.
Integrating CI Across Departments
Competitive intelligence should not remain siloed within a single department. When CI insights are shared across marketing, sales, product development, and executive leadership, the organization can leverage collective knowledge. This holistic approach encourages collaboration and ensures that everyone is informed and aligned towards common goals.
Communicating Insights Effectively
The final step in the competitive intelligence process involves sharing findings with relevant stakeholders in a manner that drives action. This requires thoughtful communication strategies, which may include:
- Dashboards: Interactive dashboards allow stakeholders to explore data in real time.
- Regular Reports: Summarizing insights and recommendations in periodic reports keeps teams updated.
- Workshops: Organizing training sessions to interpret CI and brainstorm actionable strategies can enhance understanding and engagement.
By effectively communicating insights, organizations can ensure that strategic decisions are informed by the most up-to-date and relevant information available.
Tools and Resources for Competitive Intelligence
Popular Competitive Intelligence Software
There are numerous software solutions designed to streamline competitive intelligence efforts. Some of the most popular tools include:
- Crayon: A market and competitive intelligence platform that helps track competitor changes and market trends.
- Kompyte: Offers automated competitor tracking and analytics.
- Klue: Allows teams to organize and share competitive insights easily.
The choice of tools will depend on the specific needs and budget of your organization.
Leveraging Custom Research Services
In addition to software tools, companies may benefit from partnering with third-party research firms that specialize in competitive intelligence. These firms can conduct deep-dive analyses, market assessments, and even tailored studies that suit your unique business needs. When selecting a research partner, consider their expertise, industry knowledge, and methodological rigor.
Evaluating CI Tools for Your Company’s Needs
When evaluating competitive intelligence tools, organizations should consider several factors:
- Scalability: Does the tool grow with your needs?
- User-Friendliness: Is the interface intuitive for staff?
- Integration: Does it seamlessly integrate with existing systems and workflows?
- Cost: How does the pricing align with your budget and expected ROI?
Investing in the right tools can significantly enhance a company’s ability to gather actionable intelligence and stay ahead of competitors.
Case Studies: Success Through Competitive Intelligence
Examples of Effective CI Implementation
Several companies have successfully leveraged competitive intelligence to drive growth. For instance:
- Apple: By consistently monitoring competitors in the tech space, Apple has refined its product development and marketing strategies, allowing it to maintain industry-leading innovation.
- Procter & Gamble (P&G): P&G uses competitive intelligence not just to understand competitors but also to anticipate consumer needs, which informs their product innovations.
Learning from Industry Leaders
Looking to industry leaders can provide valuable insights into best practices and effective strategies in competitive intelligence. Companies like Coca-Cola invest heavily in CI to understand consumer preferences and competitive landscape shifts, allowing them to stay ahead in the consumer goods sector.
Measuring the ROI of Competitive Intelligence Efforts
To justify investments in competitive intelligence, organizations should track the return on investment (ROI) of their efforts. This can be accomplished through:
- Performance Metrics: Establish metrics such as sales growth, market share improvements, and successful product launches linked to CI insights.
- Feedback Loops: Collect qualitative feedback from teams regarding how CI insights impacted decision-making.
By actively measuring the effectiveness of your competitive intelligence initiatives, organizations can demonstrate the tangible value that these programs provide.
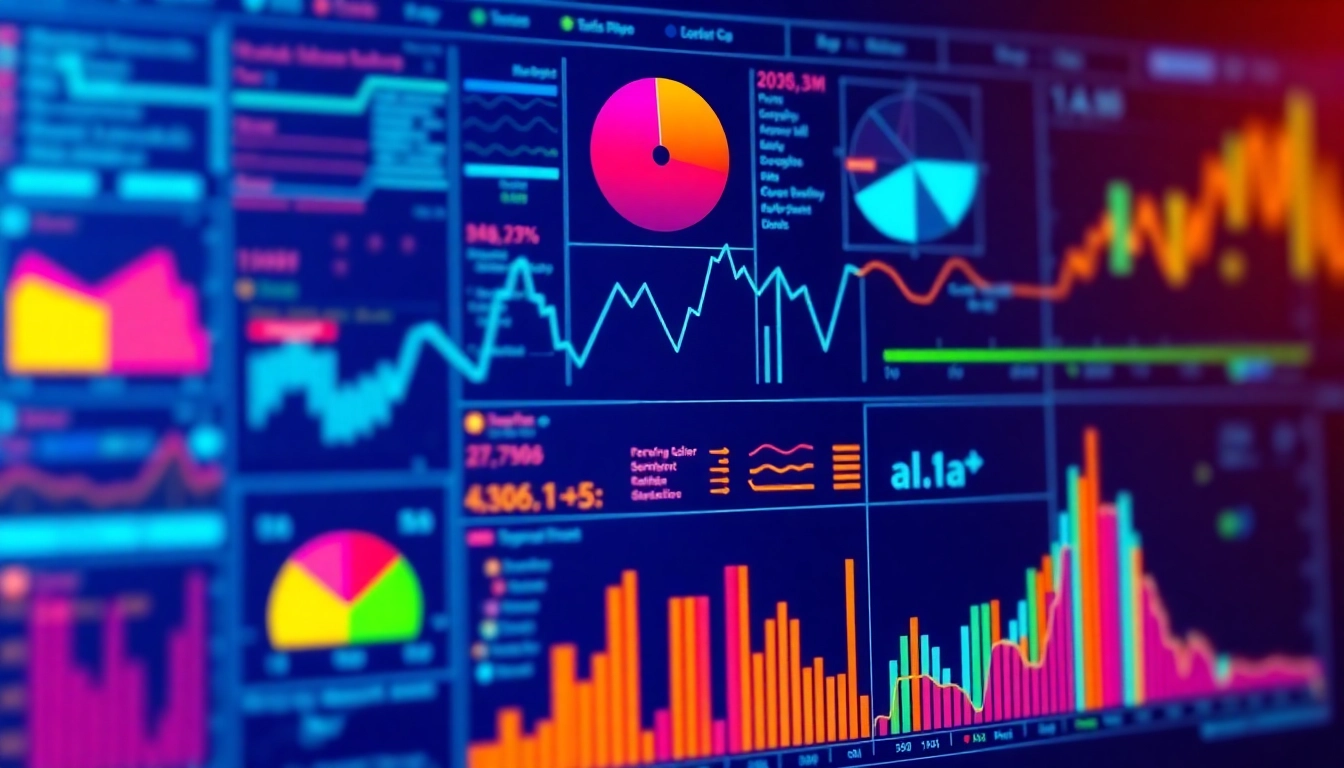
Enhancing Insights with AI Sentiment Analysis: A Comprehensive Guide
Understanding AI Sentiment Analysis
Definition and Importance
AI sentiment analysis refers to the method of using artificial intelligence technologies to interpret and classify emotions expressed in text. It operates at the intersection of natural language processing (NLP) and machine learning (ML) to extract subjective information from large volumes of data, such as social media posts, customer reviews, and feedback forms. The importance of sentiment analysis is underscored by its ability to provide organizations with actionable insights into public perceptions and emotional responses. By leveraging AI sentiment analysis, businesses can gain a strategic advantage, optimize their marketing efforts, and enhance customer relations.
How AI Sentiment Analysis Works
The process of AI sentiment analysis generally starts with data collection. Available textual data is gathered from various sources such as social media platforms, online reviews, blogs, and forums. AI models then employ natural language processing techniques to analyze this data. The analysis typically includes tokenization (breaking down text into words or phrases), part-of-speech tagging, and named entity recognition to identify key components of the text.
Once the data is pre-processed, sentiment classification can begin, with AI leveraging supervised, unsupervised, or semi-supervised learning techniques. Algorithms analyze the text through predefined rules or by learning patterns from previously labeled datasets. The end goal is to categorize sentiments into positive, negative, or neutral, providing a clear understanding of emotional tone for each piece of content analyzed.
Business Implications
AI sentiment analysis has substantial business implications. For instance, companies can gauge customer satisfaction in real-time, reacting swiftly to negative experiences. Moreover, businesses can identify trends in public sentiment towards their products or services, making it possible to tailor marketing strategies effectively.
Additionally, leveraging sentiment analysis can enhance product development cycles by interpreting customer feedback to make data-driven decisions. By examining sentiment trends over time, businesses can predict market behavior and customer needs, leading to competitive advantages.
Key Techniques in AI Sentiment Analysis
Natural Language Processing Approaches
Natural Language Processing (NLP) is the backbone of AI sentiment analysis. Techniques such as tokenization, stemming, and lemmatization play significant roles in parsing the text. Tokenization allows the system to break down sentences into individual units called tokens, which are easier to analyze. Stemming and lemmatization help normalize these tokens to their base form, improving the accuracy of sentiment analysis.
Advanced NLP approaches also involve the use of pre-trained language models like BERT (Bidirectional Encoder Representations from Transformers) and GPT (Generative Pre-trained Transformer). These models excel in capturing contextual nuances within text data, enhancing the accuracy of sentiment classification.
Machine Learning Algorithms
Machine learning algorithms play a pivotal role in sentiment analysis. Various algorithms, such as logistic regression, support vector machines (SVM), and Naive Bayes classifiers, are employed to classify sentiment accurately. More advanced methods involve deep learning techniques utilizing neural networks to uncover complex patterns within large datasets.
The choice of algorithm often depends on the size and complexity of the dataset. For extensive datasets, deep learning models are preferred due to their ability to learn from vast amounts of information and identify subtle sentiments.
Automated Sentiment Detection
Automated sentiment detection has revolutionized how businesses analyze text data by streamlining the process and allowing for real-time feedback. This automation is critical for organizations handling large volumes of information, as it drastically reduces the time and effort required for manual sentiment analysis.
Tools and platforms powered by AI enable businesses to automate sentiment detection across various channels, from customer service interactions to social media posts. Continuous learning algorithms refine sentiment analysis techniques over time based on feedback and new data, enhancing accuracy and relevancy.
Applications Across Industries
Marketing and Customer Insights
In the marketing realm, AI sentiment analysis offers profound insights into consumer behavior. By analyzing feedback across platforms, companies can develop targeted marketing strategies that resonate with their audience’s emotional responses. Understanding how customers feel about a brand can significantly influence marketing campaigns, product launches, and overall brand positioning.
Sentiment analysis can also be used to gauge customer responses to advertising campaigns, allowing companies to tweak or rethink their approaches based on public sentiment.
Product Feedback Analysis
AI sentiment analysis is essential in product management, providing companies with insights derived from customer feedback. By effectively assessing user sentiment concerning product features or usability, businesses can prioritize features in development, resolve pain points, and refine their product offerings. This strong feedback loop ensures customer expectations are met or exceeded, fostering loyalty and trust.
Social Media Monitoring
Social media represents a real-time pulse of public sentiment, making it a rich source of data for AI sentiment analysis. Companies use sentiment analysis to monitor brand mentions, measure the impact of campaigns, and track overall brand health. By understanding public emotion towards their brand, organizations can engage proactively with their audience and handle crises effectively.
Implementing AI Sentiment Analysis in Your Business
Choosing the Right Tools
When implementing AI sentiment analysis, selecting the right tools is critical for success. Various AI platforms and software offer sentiment analysis capabilities, each with its strengths. Consider factors such as ease of use, scalability, integration capabilities, and cost when choosing tools.
Popular tools include IBM Watson, Google Cloud Natural Language API, and proprietary platforms that offer custom solutions. Assessing the specific needs and technical capabilities of your business will guide you in selecting the most appropriate tool.
Establishing Best Practices
Establishing best practices in your sentiment analysis approach is vital for obtaining reliable results. Begin by ensuring data quality through proper data cleansing and pre-processing. Consistent labeling of training data is also essential for the accuracy of supervised models.
This includes defining clear criteria for positive, negative, and neutral sentiments to maintain uniformity across your data sets. Regularly updating your models with new data and feedback will ensure that your sentiment analysis remains relevant and reflective of current trends and sentiment shifts.
Measuring Performance and Impact
Measuring the performance and impact of AI sentiment analysis is crucial for demonstrating its value to your business. Key performance indicators (KPIs) such as accuracy, precision, recall, and F1 score can provide insights into model performance. Additionally, evaluating the outcomes of sentiment-based actions (i.e., marketing adjustments, product improvements) helps gauge the real-world impact of sentiment analysis initiatives.
Regular audits of sentiment analysis outcomes can also inform future strategy and help refine methods for better effectiveness over time.
Future Trends in AI Sentiment Analysis
Advancements in NLP Technology
The landscape of AI sentiment analysis is continually evolving, particularly with advancements in NLP technology. Future developments are likely to focus on improving the contextual understanding of language, enabling sentiment analysis tools to recognize sarcasm, cultural nuances, and emotional complexities more accurately.
Emerging methods in deep learning, such as transformer architectures and advanced embedding techniques, promise enhanced capabilities for understanding and interpreting user sentiment across diverse sectors.
Ethical Considerations
As with many AI technologies, ethical considerations in sentiment analysis are paramount. Issues surrounding privacy, data security, and the potential for misuse must be addressed. Organizations should strive for transparent practices regarding data usage and be clear with customers about how their information is analyzed.
Adopting ethical frameworks that guide the development and use of sentiment analysis technologies can help promote responsible AI usage and build public trust in these systems.
Integration with Other AI Applications
Integrating sentiment analysis with other AI applications will propel its capabilities even further. Combining sentiment analysis with chatbots and customer service platforms can enhance user interaction quality, providing responses based on individual customer sentiment.
Moreover, integrating sentiment analysis with predictive analytics can allow businesses to foresee market trends and changes in consumer sentiment, enabling proactive decision-making.
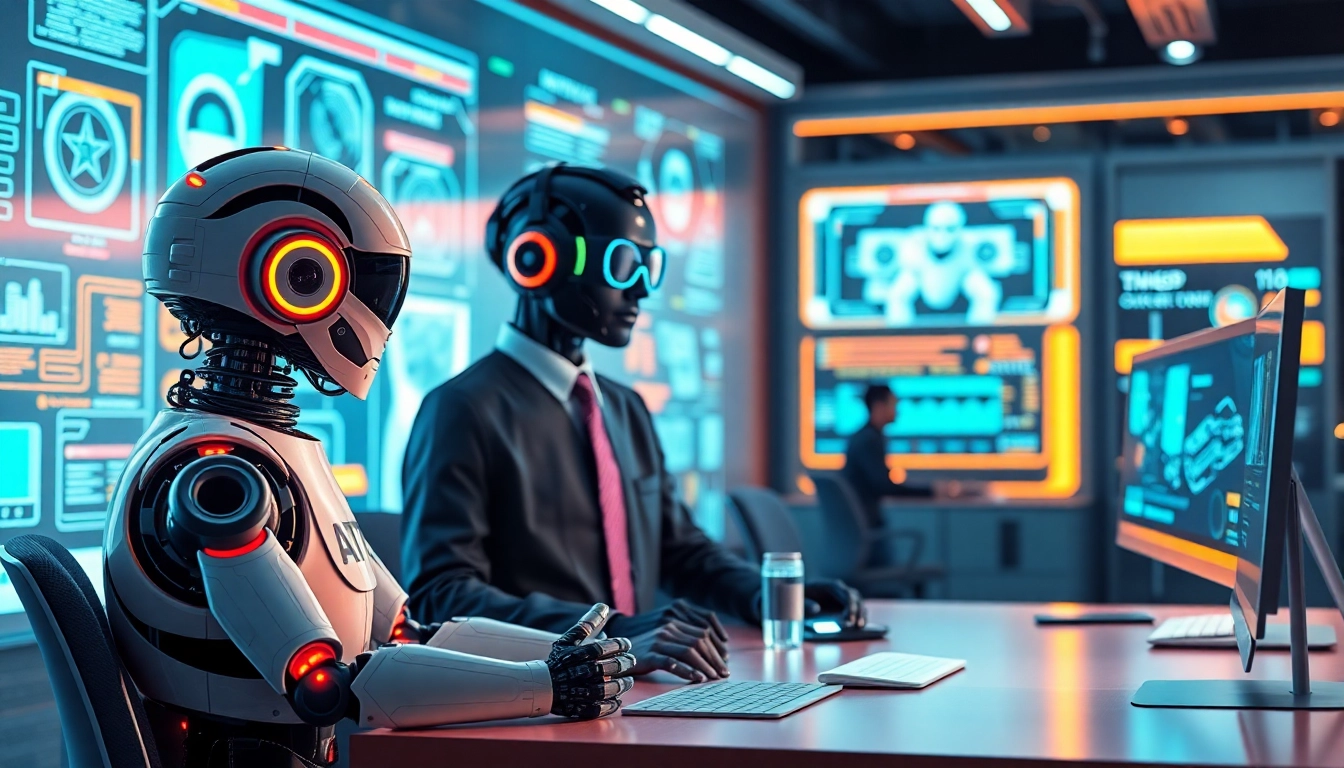
How Agentic Human AI is Redefining Work and Collaboration in Today’s World
Understanding Agentic Human AI
Defining Agentic Human AI
Agentic Human AI refers to advanced artificial intelligence systems designed to perform tasks autonomously, making decisions and taking actions without continuous human oversight. These AI systems leverage machine learning, natural language processing, and other advanced technologies to understand context, grasp complex patterns, and drive productivity in various domains. In essence, Agentic Human AI signifies a paradigm shift where AI not only assists humans but can operate independently across multifaceted environments.
Historical Context and Evolution
The evolution of Agentic Human AI can be traced back to early AI systems that relied heavily on rule-based algorithms. As computational power increased and vast data became more accessible, machine learning techniques emerged, allowing AI systems to improve their performance based on experience. The introduction of neural networks led to breakthroughs in understanding complex data structures, paving the way for the development of autonomous agents capable of acting with a degree of agency.
Key milestones in this evolution include:
- 1950s-1970s: Early AI research focuses on symbolic AI, where machines follow explicit rules to solve problems.
- 1980s-1990s: The rise of machine learning, particularly supervised and unsupervised learning, which enabled systems to learn from patterns in data.
- 2000s-Present: Advancements in deep learning and the emergence of big data, leading to sophisticated neural networks that operate autonomously.
Key Characteristics of Agentic AI
Agentic Human AI is characterized by several defining features that differentiate it from traditional AI systems:
- Autonomy: These systems can operate independently, processing information and making decisions without needing constant human input.
- Adaptability: They can learn and adjust their strategies based on changing circumstances and new data.
- Contextual Awareness: Agentic AI systems possess the capability to understand their environment and surroundings, allowing for more informed decision-making.
- Self-Optimization: They continually improve their performance by analyzing past outcomes and adjusting their approach accordingly.
Working Mechanisms of Agentic Human AI
Autonomy in Decision-Making
The hallmark of Agentic Human AI is its ability to make decisions autonomously. This capability arises from sophisticated algorithms that analyze vast datasets to identify trends and predict outcomes. For instance, in business applications, agentic AI can optimize supply chain logistics by predicting demand fluctuations and adjusting inventory levels without human intervention. This not only saves time but also minimizes errors that could occur through human oversight.
Data Utilization and Learning
Data is the lifeblood of any AI system, and Agentic Human AI is no exception. These systems employ advanced data analytics to gather, process, and learn from data streams continuously. Using techniques such as reinforcement learning, they adapt based on real-time feedback from their environment. This iterative learning process enables them to refine their approaches over time and develop strategies that are more effective and efficient.
Interactivity with Human Users
Despite their autonomy, Agentic Human AI systems are designed to interact with human users seamlessly. They utilize natural language processing to communicate effectively, understand user queries, and provide relevant information or assistance. For example, in customer service applications, these AI systems can handle inquiries and resolve issues independently, escalating complex cases to human agents only when necessary.
Applications of Agentic Human AI
In Business and Work Environments
Agentic Human AI is already transforming the business landscape by automating routine tasks and providing insights that were previously unattainable. In sectors such as finance, marketing, and operations management, AI systems are enhancing decision-making processes and driving efficiencies.
Examples of applications include:
- Financial Services: AI-driven algorithms can analyze market trends, recommend investments, and even execute trades autonomously, reducing human error and optimizing financial performance.
- Marketing Automation: AI systems can analyze consumer behavior patterns to create targeted campaigns, optimizing engagement, and improving conversion rates.
- Human Resources: AI can streamline recruiting processes by screening resumes and matching candidates to job descriptions, allowing HR teams to focus on more strategic initiatives.
Healthcare Innovations
In healthcare, Agentic Human AI holds the potential to revolutionize patient care and clinical decision-making. By analyzing patient data and medical research, AI can assist healthcare professionals in diagnosing conditions and recommending treatment plans.
Notable applications include:
- Diagnostic Tools: AI algorithms can analyze medical images, such as X-rays and MRIs, identifying anomalies with high accuracy.
- Personalized Medicine: AI can help tailor treatments based on individual patient data, improving treatment efficacy and minimizing adverse effects.
- Virtual Health Assistants: These AI systems can engage with patients, monitor symptoms, and provide timely interventions, enhancing the overall healthcare experience.
Educational Tools and Resources
Education is another domain where Agentic Human AI is making significant strides. AI-based educational tools can tailor learning experiences to individual student needs, offering personalized support that enhances engagement and knowledge retention.
Applications in education include:
- Adaptive Learning Platforms: These systems adjust content delivery based on student performance, ensuring a customized learning path that addresses knowledge gaps.
- AI Tutors: AI-powered tutoring systems can provide assistance on demand, helping students grasp complex concepts at their own pace.
- Administrative Efficiency: AI can automate administrative tasks such as grading and scheduling, allowing educators to focus more on teaching and mentoring.
Challenges and Ethical Considerations
Technological Limitations
Despite its potential, Agentic Human AI faces several technological challenges. One significant hurdle is the need for high-quality, unbiased data. AI systems are only as effective as the data they are trained on, and any bias in the data can lead to skewed outcomes. Additionally, ensuring data privacy and security remains a critical concern, particularly in sensitive areas like healthcare and finance.
Ethical Implications
As Agentic Human AI systems increasingly operate autonomously, ethical considerations become paramount. Questions surrounding accountability, transparency, and the potential for misuse arise. For example, if an AI system makes a harmful decision, determining responsibility can be complex. Ethical guidelines and frameworks must evolve alongside technological advancements to mitigate risks and ensure responsible AI use.
Future Regulations and Guidelines
In light of these challenges, regulators worldwide are beginning to explore frameworks that govern the use of AI technologies. Future regulations may mandate stringent testing protocols for AI systems, establish guidelines for ethical conduct, and promote transparency in AI decision-making processes. Collaborative efforts between governments, tech companies, and civil society will be essential to develop effective regulatory strategies that balance innovation with societal welfare.
The Future of Agentic Human AI
Potential Developments on the Horizon
The future of Agentic Human AI is poised for remarkable advancements. Innovations in quantum computing and neuromorphic chips may significantly enhance the capabilities of AI systems, making them even more powerful and efficient. Additionally, the integration of AI with other technologies, such as blockchain and IoT, could lead to groundbreaking applications across various sectors.
Impact on Workforce Dynamics
As Agentic Human AI continues to evolve, its impact on the workforce is likely to be profound. While AI will automate certain tasks, it will also create new roles that require human oversight, creativity, and emotional intelligence. Upskilling and reskilling initiatives will become essential as organizations adapt to this new landscape, ensuring that workers are equipped to thrive alongside AI technologies.
Long-term Vision for Society
Ultimately, the long-term vision for Agentic Human AI is one where these systems enhance human potential rather than replace it. By automating repetitive tasks and providing insights, AI can free individuals to focus on more creative, strategic, and fulfilling endeavors. A collaborative relationship between humans and AI can lead to innovative solutions that address pressing global challenges, fostering a future where technology and humanity coexist harmoniously.
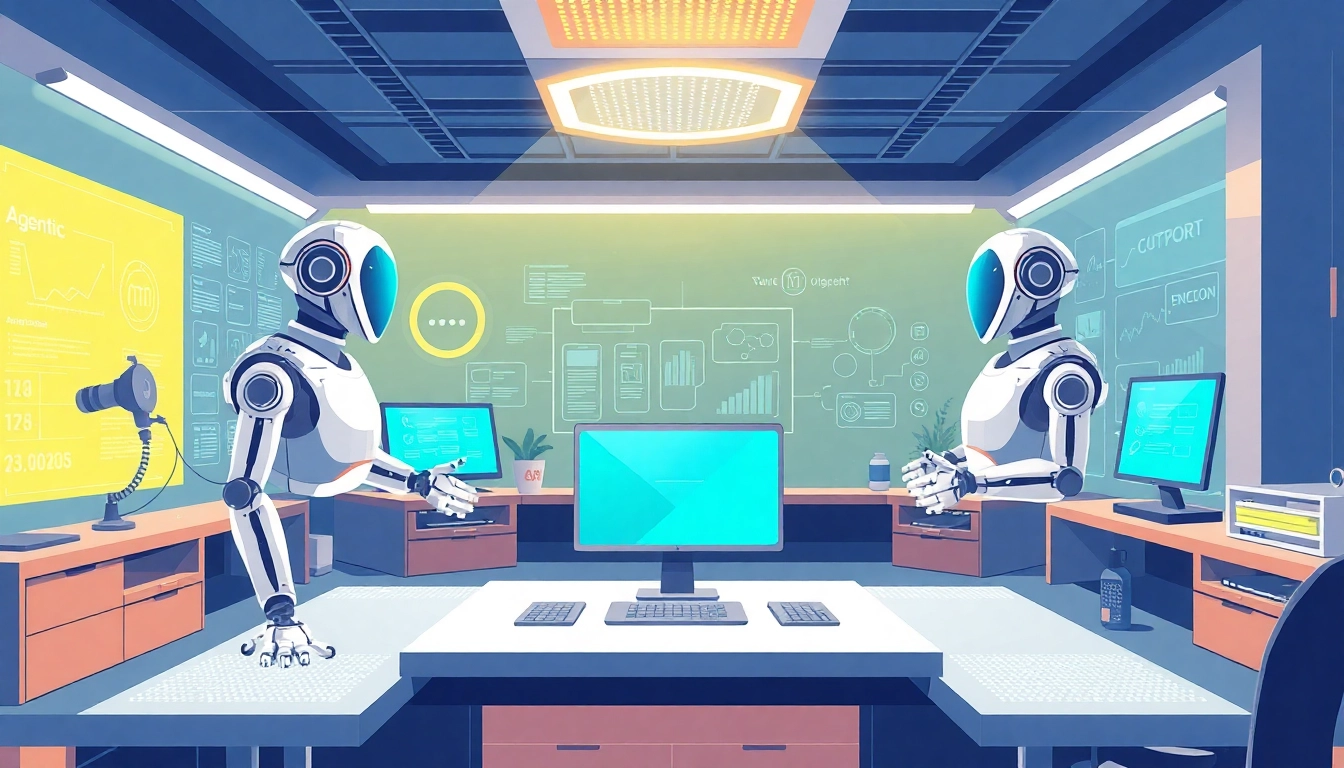
Harnessing Agentic Human AI: Transforming Autonomous Decision-Making in Modern Workspaces
Understanding Agentic Human AI
In the fast-paced world of artificial intelligence, the emergence of Agentic Human AI represents a significant stride towards developing autonomous systems capable of sophisticated decision-making. This innovative paradigm of AI transcends traditional machine learning models by empowering AI agents to not only process data but also act independently to achieve specific goals. This article delves into the definition, characteristics, historical context, and the transformation from conventional AI systems to agentic ones.
Definition and Key Characteristics
Agentic Human AI refers to AI systems endowed with a degree of agency—meaning they can autonomously execute tasks and make decisions without continuous human oversight. These systems are designed to analyze their environments, interpret data, set objectives, and choose actions based on those objectives. Key characteristics of agentic AI include:
- Autonomy: Agentic AI can operate independently, engaging in tasks without requiring human intervention.
- Adaptability: These systems can adapt to new information or changing conditions, allowing them to refine their actions and strategies over time.
- Goal-oriented Behavior: Agentic AI is programmed to perform specific tasks aimed at achieving defined goals, whether in data analysis, decision-making, or operational tasks.
- Complex Problem Solving: Utilizing advanced algorithms, agentic AI is capable of solving intricate, multi-step problems that may be beyond traditional AI’s capacity.
Historical Context and Evolution
The concept of agentic AI is rooted in decades of advancement within the field of artificial intelligence. Initially, AI systems were primarily rule-based, executing tasks as predefined by human programmers. This limited approach restricted flexibility and adaptability. With the advent of machine learning and neural networks, AI began to learn from data, but human oversight was still necessary.
As computational power surged and data availability expanded, researchers began exploring AI models that could learn autonomously and make decisions grounded in probabilistic reasoning. This laid the groundwork for the agentic models we see today. In recent years, developments in reinforcement learning and other advanced methodologies have propelled agentic AI, bringing it closer to operational real-world applications across diverse industries.
Comparison with Traditional AI Systems
To appreciate the advancements brought by agentic AI, we must compare it with traditional AI systems. Unlike traditional models that generally require explicit programming and continuous human monitoring, agentic AI embodies a paradigm shift by incorporating cognitive functions akin to human reasoning. Here are some notable distinctions:
- Decision-Making: Traditional AI systems rely heavily on pre-programmed rules, whereas agentic AI utilizes dynamic decision-making capabilities based on live data and context.
- Human Interaction: While traditional AI often necessitates human input for analysis or execution, agentic AI can operate independently, significantly reducing the need for human oversight.
- Adaptability: Agentic AI systems use feedback loops to adjust their behaviors and strategies on the fly, compared to the static nature of traditional algorithms, which require reprogramming for changes.
Applications of Agentic Human AI
The versatility of agentic AI allows for its applications across various sectors, from healthcare to finance, education, and beyond. Below, we examine some notable area implementations, backed by case studies and future potential.
Industry Implementations
One of the most compelling features of agentic human AI is its adaptability across various industries. Here are a few domains actively integrating this technology:
- Healthcare: Agentic AI is revolutionizing diagnostics and patient care by autonomously analyzing medical images and patient data to suggest treatment plans, enhancing the precision and speed of healthcare delivery.
- Finance: Financial institutions utilize agentic AI to detect fraud, optimize trading strategies, and assess creditworthiness. These systems can analyze vast datasets in real-time to make decisions that can lead to significant financial savings and improved compliance.
- Manufacturing: In the industrial sector, agentic AI can oversee production lines, predict machine failures, and optimize supply chain management, thereby increasing efficiency and minimizing downtime.
Case Studies and Success Stories
Numerous companies have harnessed the power of agentic AI to propel their operations forward:
- Healthcare Innovations: A healthcare startup developed an agentic AI platform that successfully diagnoses skin cancer based on image recognition, achieving an accuracy rate higher than most dermatologists.
- A Financial Breakthrough: A leading bank implemented an agentic AI system that accurately forecasted market trends, leading to a 20% increase in investment portfolio performance within a year.
Future Potential Across Sectors
The future implications of agentic AI are vast. As technology evolves, we expect to see synergies between AI agents and existing technologies, leading to enhanced efficiencies and capabilities. Future developments may include:
- Widespread use in sustainable practices, such as smart grid management and resource optimization.
- Collaborative robots (cobots) in manufacturing and service industries that work alongside humans while autonomously completing specialized tasks.
Benefits and Challenges
The embrace of agentic AI presents several advantages and challenges that organizations must navigate to fully leverage this technology.
Advantages of Implementing Agentic Human AI
Organizations that successfully integrate agentic AI can realize significant benefits:
- Increased Efficiency: By automating routine tasks and decision-making, agentic AI allows human workers to concentrate on more strategic initiatives, improving overall productivity.
- Enhanced Decision-Making: Agentic AI draws from real-time data to inform decisions, resulting in more informed and timely action.
- Cost Savings: Implementing agentic AI can reduce operational costs by minimizing human error and streamlining processes.
Common Challenges and Mitigation Strategies
Despite the advantages, organizations may encounter challenges including:
- Integration Difficulties: Merging new agentic AI systems with existing technologies can be complex. A phased rollout approach, supplemented with robust training programs, can ease this transition.
- Data Privacy Concerns: Handling sensitive data must be prioritized to maintain compliance with regulations such as GDPR. Organizations should implement strict data governance frameworks and encryption protocols.
Ethical Considerations in Autonomous Systems
As AI systems gain autonomy, ethical concerns arise around accountability, bias, and job displacement. It is crucial for organizations to establish ethical guidelines governing AI deployment, ensuring systems remain transparent, fair, and accountable for their actions.
Integration Strategies
Successful integration of agentic human AI into existing business frameworks requires thoughtful planning and execution. Here are key strategies to consider:
Steps to Incorporate Agentic Human AI into Your Business
The incorporation of agentic AI into your business can be navigated using the following steps:
- Assess Needs: Identify areas within your operations that could benefit from automation or better decision-making.
- Select Appropriate Tools: Choose AI platforms and tools that align with your strategic goals and operational needs.
- Pilot Testing: Conduct pilot studies to evaluate performance, gather feedback, and make adjustments before full implementation.
Interoperability with Existing Technologies
Ensuring that agentic AI systems seamlessly integrate with your existing technology stack is vital for operational cohesion. Companies should prioritize systems that offer interoperability features, allowing for data exchange and multi-system collaboration.
Monitoring and Governance Best Practices
Establishing a robust governance framework for agentic AI systems is essential for ongoing performance management:
- Regularly audit AI decisions to ensure they align with business objectives and ethical guidelines.
- Maintain transparency in how AI models operate, keeping stakeholders informed about potential biases and data sources.
The Future of Agentic Human AI
Looking ahead, the trajectory of agentic human AI promises to be transformative across various aspects of society and industry. Here, we explore anticipated developments and the evolving landscape of human-AI collaboration.
Trends and Predictions in AI Development
As technological capabilities expand, we foresee trends such as:
- A growing emphasis on ethical AI, integrating compliance and ethics seamlessly into AI systems.
- Increased collaboration among AI and humans, leveraging the strengths of each to achieve enhanced outcomes.
Continuous Learning and Adaptation
Ongoing advancements in machine learning will continually enhance agentic AI’s capability to learn from past experiences, adapt effectively to changes, and maintain relevance in dynamic environments.
Collaboration Between Humans and AI
Finally, the future of work will increasingly hinge on collaboration between agents and humans. As agentic AI systems take on more responsibilities, human workers will shift into roles that capitalize on creativity, empathy, and strategic thinking—qualities inherent to human nature.
In conclusion, the evolution of agentic human AI marks a pivotal chapter in technological advancements, poised to reshape industries and redefine human interactions with machines. Organizations that embrace this paradigm will not only enhance their operational capabilities but also position themselves at the forefront of innovation.